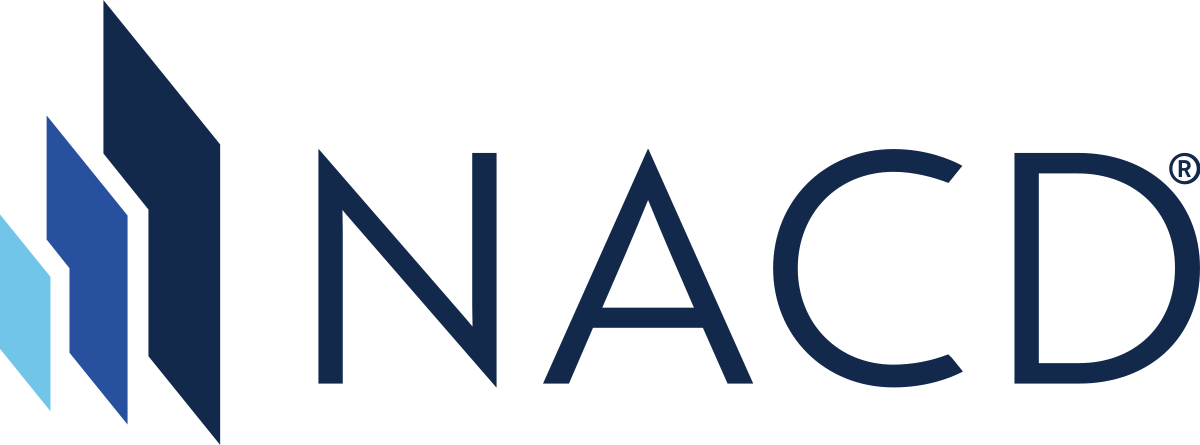
Trending Oversight Topics
Governance Surveys
Center for Inclusive Governance
How Boards Can Oversee AI Strategy
12/07/2023
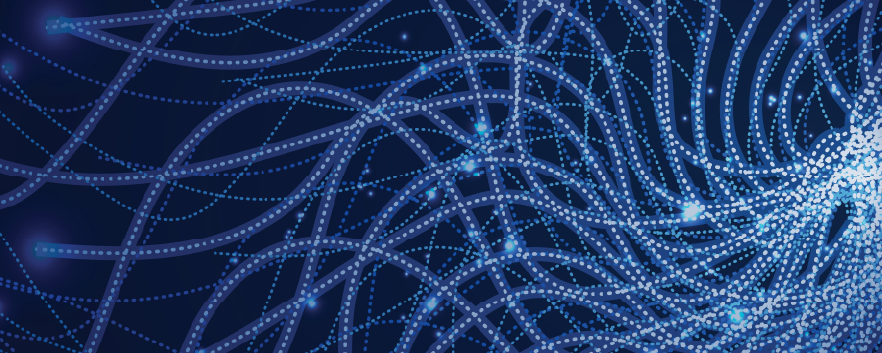
Deploying AI might be risky—but not as risky as not deploying it at all.
Imagine your organization’s artificial intelligence (AI) strategy as riding a descending escalator; standing still means falling behind. Adversaries are infamously early adopters of new technologies, so constant effort is required to keep up.
Most board conversations involving AI focus on risk. While there are inherent risks associated with AI (e.g., data leakage, intellectual property (IP) loss, and its use by cybercriminals), a single-minded focus on adoption risks ignores a greater risk: the risk of non-adoption.
If AI integration is forced, how can companies deploy it responsibly? It begins with understanding how AI adoption will affect strategy and capital allocation, the path to building internal AI expertise, and, finally, how AI-related risk can best be managed.
How will AI shift business strategy?
In our digital age, every company is a software business. Businesses successful in capitalizing AI can become more customer-centric and frictionless to do business with and can capitalize on data-driven insights to improve processes and performance, but they must incorporate security and service assurance.
Because AI democratizes creativity, exploiting its benefits requires flexibility. This may mean responding to new competitors entering your business vertical or it may mean more goods or services are in play as offerings. Be mindful of these developments’ strategic impact on business operations.
How will AI impact capital allocation?
AI requires a long-term financial commitment to people, education, resources, and platforms. AI platforms are data-hungry and capital-intensive because of the required processing power and energy needed to drive. Turning organizations’ data into uniform input intelligible by AI algorithms is tedious, time-consuming, and expensive. However, it is an essential step when developing a working AI platform.
Companies must be willing to take risks and experiment with AI and big data. They need to understand that failure is a part of experimentation.
How will we build our AI knowledge base?
Enhancements in AI competence should be included in board and executive succession plans. This requires comprehensively up-skilling leadership. AI know-how can be thought of as a three-legged stool, unstable without each supporting leg, propped up by the following:
- Domain knowledge: Broad business experience can help in determining the value AI presents. These insights determine where AI can advance strategic initiatives and require thinking critically through potential pitfalls that could prevent success.
- Process knowledge: Process specialists will design the experiment from start to finish. These individuals identify data needed, data the organization possesses, and how to reconcile the two. The process specialist chooses the tools and ensures initial results align with the intended outcome.
- Information technology (IT) knowledge: IT specialists must then run the tools, troubleshoot, and lay technical foundations for pulling information from tools such as enterprise resource planning and customer relationship management systems. Without this plumbing, AI enablement is just a nice idea.
How can boards oversee AI related risk?
Understanding AI related risk requires grasping how bad actors use it to carry out successful attacks. For example, most know that AI can help automate and polish attacks to create more convincing social engineering pretexts.
A more severe and novel risk involves organizations embracing AI to gain a competitive advantage while failing to put guardrails in place. The Samsung incident is an instance of (likely) well-intentioned employees entering proprietary IP into a generative AI tool for productivity’s sake. This IP then becomes part of the model, not just the originating company.
It’s possible that the leaked IP will become lost among millions of other inputs. But, in cutting-edge applications, it could form the model’s foundational understanding of a topic and hence feature in most of its responses to questions concerning it. Organizations then risk their once tightly controlled IP becoming common knowledge and losing patents.
This pinpoints an issue for generative AI engines: everyone wants the benefits of a model trained on new insights, but no company wants to relinquish control of its data. This is considered the first-mover disadvantage as other companies may benefit from the research and development your company developed without any guarantee of being similarly compensated.
Additional, specific risks arise from these activities:
- Employees using personal devices and corporate data to solve business problems with public large language models (LLMs) such as ChatGPT.
- Internal deployment of LLMs sourcing data from confidential sources being “made available” to people who should not have access.
- Using a combination of private and public LLMs without understanding how internal information is being used publicly.
- Using a combination of indexing and LLMs that don’t honor entitlements or show a search entry to someone who shouldn’t be able to see it.
Boards should participate in the creation of the AI governance and policy framework and ensure that the controls in the framework exist, have people aligned with them, and can be evidenced by means of an internal audit. Additionally, boards should think about red-teaming approaches for AI. Boardroom AI risk discussions should be incorporated into overall cyber-risk oversight practices.
Balancing Our AI Embrace
While AI can augment human intelligence and accelerate productivity, critical thinking will still fall to humans for some time to come.
Board members should increase their AI understanding of consequential security risks. To do this, leadership must start by identifying the experiments that can prove the business case for AI implementation, carefully planning for its success, and ensuring the expertise to execute successfully. This means understanding the process architecture, roles, and work products, as well as identifying where the use of AI-enabled tools will significantly improve speed, quality, and completion.
Zscaler is a NACD partner, providing directors with critical and timely information, and perspectives. Zscaler is a financial supporter of the NACD.
Helmuth Ludwig, NACD.DC, is a professor of practice for strategy and entrepreneurship at Southern Methodist University. He previously served as the chief information officer for an international conglomerate and currently sits on multiple boards. Ludwig is a coauthor of a new book on board-level cyber-risk management titled, Cybersecurity: Seven Steps for Boards of Directors.
Andrew Brown is a seasoned enterprise technology executive with more than 30 years of hands-on experience running large organizations in banking, telecommunications, oil, and pharmaceuticals. He has served as a member of the Zscaler board of directors since October 2015 and is the CEO of Sand Hill East. Brown is a coauthor of a new book on board-level cyber-risk management titled, Cybersecurity: Seven Steps for Boards of Directors.